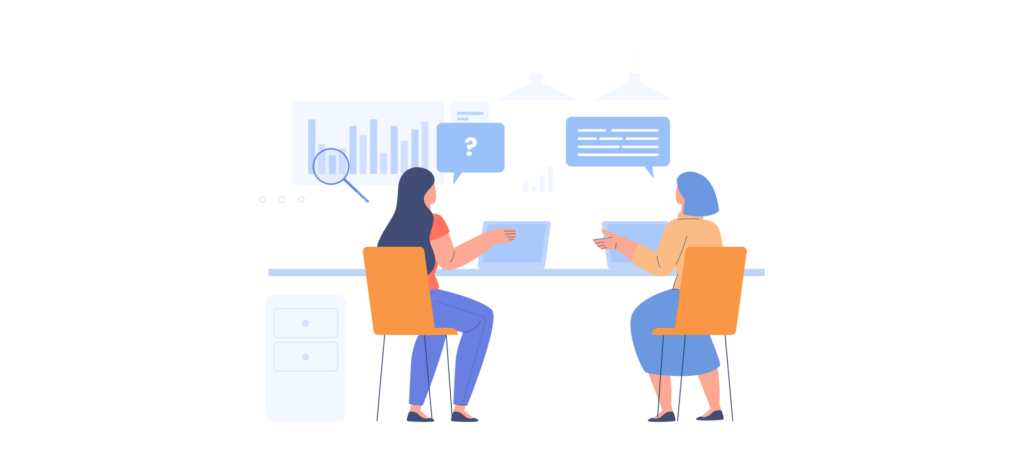
I had the opportunity to do my seven-week internship at Data Talks, a Saas company that offers a customer data platform (CDP). They help businesses collect, analyze, and act on their data to create a world-class customer experience. Since I am a digital analytics student, I found Data Talks and their product really interesting. Thus, I hoped by doing my internship with them to gain more knowledge on data and analysis.
The goal of my internship was to gain practical experience in digital analytics using different analytics tools and get an understanding of what it’s like to work as a digital analyst. Some of my main tasks were making a measurement plan for the company and measuring website traffic in Google Analytics and other conversion points throughout the customer journey. Another important task was to create an attribution model based on Google Analytics data and Marketing Automation software. I was very excited to do my internship at a company that works data-driven and to learn how a Customer Data Platform (CDP) works.
Before we dive right in...
Subscribe to our blog today to ensure that you never miss valuable posts such as this one. We are passionate about helping sports organizations deliver a world-class fan experience, because better fan experience means better business. So why not use this opportunity to the fullest?
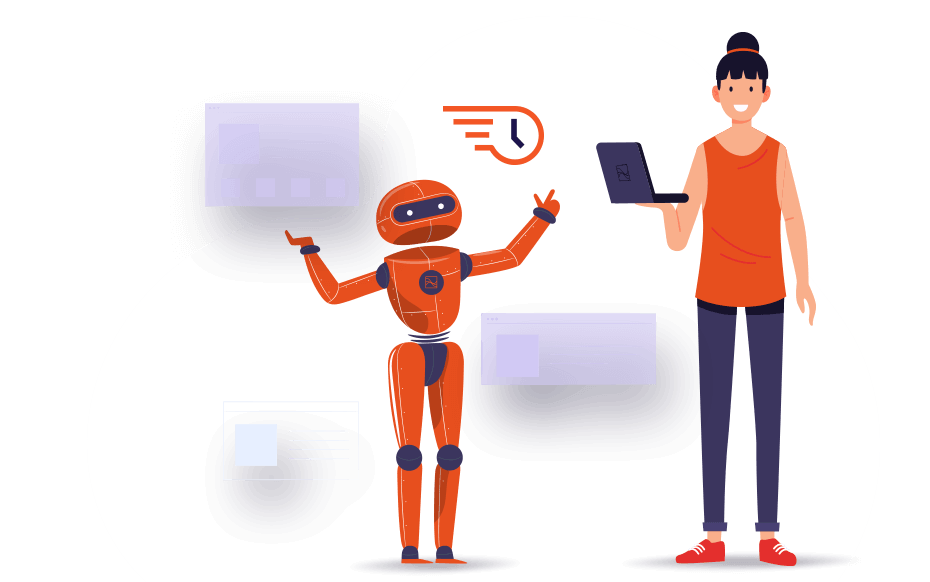
What is a CDP?
A Customer Data Platform (CDP) is software that collects and organizes customer data across various touchpoints. CDPs aggregate and structure real-time data into individual, centralized customer profiles. Data Talks CDP is categorized as a data & analytics CDP. It collects and analyzes customer data from scattered sources into one place and then offers a 360° customer view where you can discover valuable insights for your business. You can quickly act on your insights using Data Talks automated campaigns to create more relevant, personalized marketing and create an outstanding customer experience.
What did I do during my internship?
After a proper onboarding and solid introduction of their CDP, I was ready to start creating a measurement plan. This document would later guide me when building and comparing attribution models in Google Analytics.
Creating a Data Talks Measurement Plan
A measurement plan is a document that outlines business objectives and specific goals/ tactics and metrics to measure success and determine which areas need extra focus. It provides a structure that will help you implement strategies and measure results. It provides a framework and is a good way to report all the information related to your marketing efforts so you can see if the objectives are being met.
During my internship, Data Talks was on a shifting journey into a product-led growth strategy, and my measurement plan was focused on this strategy. Product-led growth (PLG) is a business methodology in which user acquisition, expansion, conversion, and retention are driven primarily by the product itself. In the measurement plan I made, it was essential to set up correct KPI’s that collect the right information for the tactics that were set up for this strategy.
KPIs are a set of key indicators that help your company assess progress toward strategic objectives, and they allow you to track how well they are doing to meet your objectives. Once the measurement plan and all the strategies and tactics were stated, I could start with making and comparing two types of attribution models.
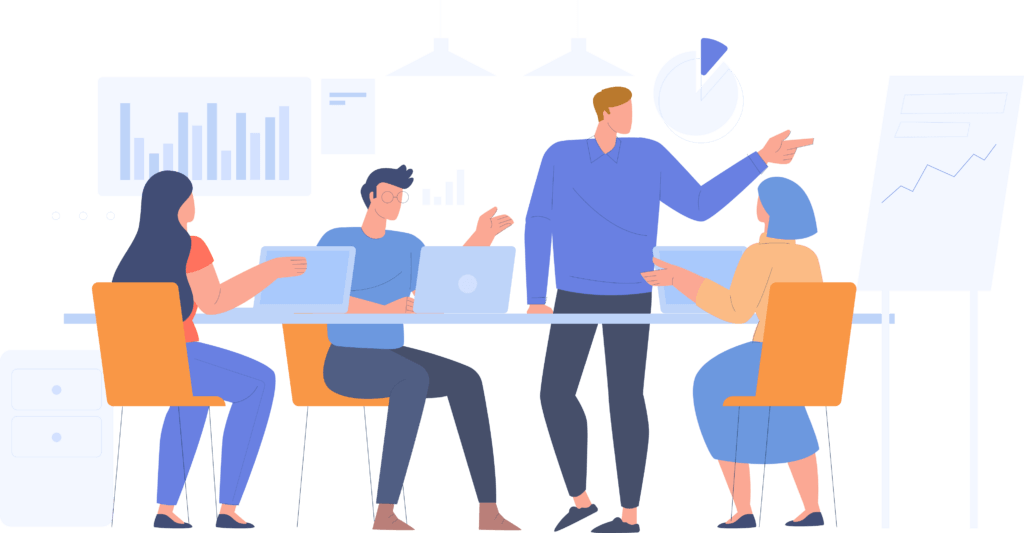
Comparing two attribution models for Data Talks
Attribution modeling is a framework for analyzing which touchpoints, or marketing channels, receive credit for a conversion. Each attribution model distributes the value of a conversion across each touchpoint differently. By comparing multiple attribution models, it’s easier to understand how different marketing channels work together to generate conversions, so you can assign a conversion value to each channel.
The basic premise of multi-touch attribution is that all touchpoints in a customer journey impact conversion and the question is: how much of an impact does each of them have?
Multi-touch attribution suits B2B companies because of the complexity of modern customer journeys. All touchpoints contribute, in one way or another, to a conversion. At Data Talks, I was assigned 2 different attribution models: The position-based and the time-decay attribution models.
The position-based attribution model gives credit to those touchpoints that depend on their position. The credit for a sale is split between a prospect’s first interaction with your brand and the moment they convert into a lead. 40% of the credit is given to each of these points, while the remaining 20% are dispersed across any other touchpoints in the middle.
When to choose the position-based attribution model:
Position-based attribution is a robust model for many business types that have multiple touchpoints prior to a conversion. It gives at least some credit to every interaction. But, it awards more weight to the two most the first time a customer found you and the interaction that prompted a conversion.
The time-decay attribution model is a multi-touch attribution that spreads out the value across multiple events, and it also takes into consideration when each touchpoint occurred. Interactions that occur closer to the time of purchase have more value attributed to them. The first interaction gets less credit, while the last interaction will receive the most.
When to choose time decay attribution:
If relationship-building is a significant factor in a business’s success, time decay attribution can be a helpful model. This model would be suitable for when you are dealing with particularly long sales cycles.
After setting up the attribution modeling in Google Analytics and pulling out some data from social media, I could analyze and compare which model would suit Data Talks better. There was not as much data available as I would like, but just enough to see that these attribution models I was comparing were very similar in crediting conversion points for this kind of Saas company.
Results
My conclusion was that Data Talks would need different approaches for different channels. As for a Saas company, comparing these two attribution models, both would be suitable – with Time decay attribution being the preferred one. There are too many touchpoints in Data Talks’ customer journey, and they all have an impactful role since the conversion paths often don’t move in a straight line. Therefore, a suggestion would be to set up different attribution models for the website, social media, and email since different channels have different purposes for Data Talks. For social networks, especially LinkedIn, time decay or position-based attribution would suit best. For emails, the last non-direct attribution brings the most conversions. For the Data Talks website, linear attribution that splits credits for the conversion equally between all interactions the customer had with the company would give a more balanced look and understanding of the whole marketing strategy for the website.
Final thoughts
During my internship, there were a few difficulties that I stumbled upon at the beginning. Before starting with the attribution modeling, I discovered some implementation errors in Google Analytics, and the test view and the master view had some issues, but these were easily corrected. The lack of proper goal setup made it a bit more challenging to analyze the attribution models as it resulted in some limited data amount, but I was able to work with that anyway.
Overall, I am happy with my internship at Data Talks, where I got to be a part of a great team. The internship was well organized, and I got to practice reporting, visualization, and other technical implementations.
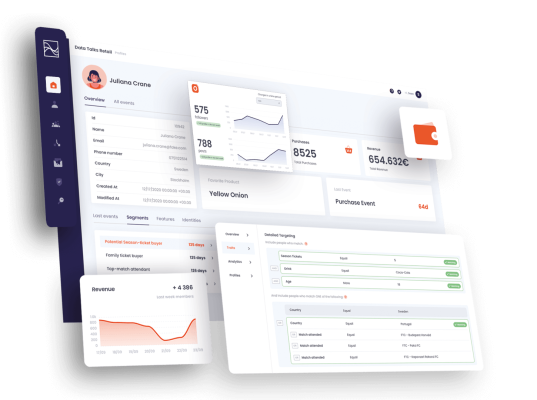